Yong-Min Shin
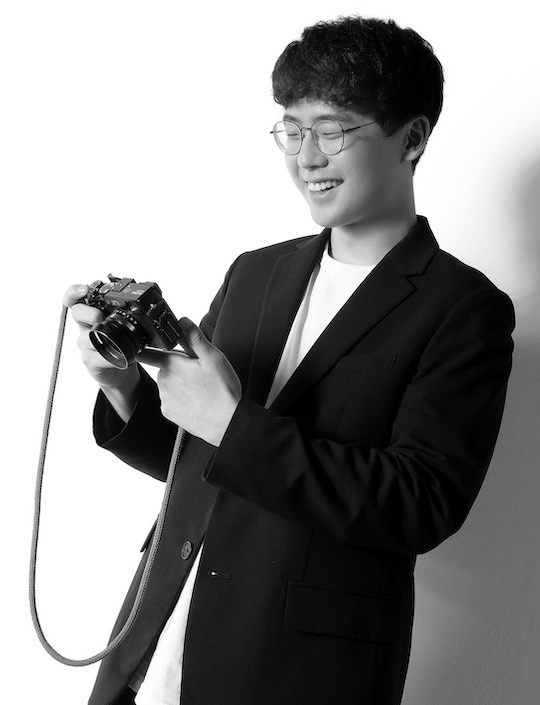
jordan3414@yonsei.ac.kr
Hello! I am a Ph.D candidate in MIDaS Lab led by Prof. Won-Yong Shin, Dept. Computer Science & Engineering in Yonsei University, South Korea. Before joining MIDaS, I graduated from Yonsei University in Feb. 2019 as a physics major.
My primary interest is to find elegant and simple solutions to interesting and important problems on graph data and graph neural networks (GNNs). More specifically, my main research topics have been focused primarily on…
- Explainability in graph neural networks
- Efficient graph learning
And my current research focuses on…
- Exploiting explanations from graph learning modules to improve the underlying data
- Diving into the evaluation protocols for explainability, especially using attention weights
- Fusion of graph neural networks with large language models
I also have research experiences in…
- Time-series with INR
- Graph representation learning
- Explainable healthcare
- Recommendation systems
…which are published in conferences like AAAI, IJCAI, SIGIR, LoG, and journals like PAMI, PlosOne, and so on.
I am always interested in reading about for other research fields, for example in…
- Mechanistic Interpretability: I personally think that this is the future of XAI research. I just started to pick up some papers on this topic and I am trying to understand the concept of mechanistic interpretability. As a means to learn more about this topic, I am currently translating several important articles on mechanistic interpretability into Korean. Check out this Gitbook if you are interested!
- Causal Inference: With the help of Brady Neal’s Introduction to Causal Inference, I am trying to understand the concept of causality to understand how it can impact the explainability of machine learning models.
I am always open to new ideas and collaborations, so feel free to reach out to me!
Here is my formal CV :)
And I also have a personal blog. Check it out!
News
Jun 23, 2025 | I started a new position as a research scientist intern at LG AI Research in Seoul, South Korea, from June 2025. I will be working on applying machine learning methods to chemical and material science problems, and I am excited to contribute to the research in this area. I am grateful for the opportunity and look forward to collaborating with the LG AI Research team. |
---|---|
Dec 10, 2024 | The paper “Faithful and Accurate Self-Attention Attribution for Message Passing Neural Networks via the Computation Tree Viewpoint” was accepted in AAAI 2025 where I am the first author. Thanks to Prof. Shin for the supervision and to the reviewers! The paper is available here. See you in Philadelphia! |
Jul 29, 2024 | The extended version of my LoG paper “Propagate & Distill: Towards Effective Graph Learners Using Propagation-Embracing MLPs” has been accepted to the journal Knowledge-Based Systems (title: “Unveiling the unseen potential of graph learning through MLPs: Effective graph learners using propagation-embracing MLPs”). Thanks to Prof. Shin for the supervision and to the reviewers! The paper is available here as well as the arXiv version. |
Jun 5, 2024 | The paper “On the Feasibility of Fidelity\(^{-}\) for Graph Pruning” was accepted in IJCAI 2024 Workshop on Explainable Artificial Intelligence (XAI) where I am the first author. Thanks to Prof. Shin for the supervision and to the reviewers! The paper is available here. |